27 Dec 2020
Disclaimer: Although I currently work at NVIDIA, I wrote this article before I joined in July 2021. All opinions in this post are my own and not associated with my company.
Over the past few months or so, I've had to work on getting pretrained deep learning (DL) models downloaded off GitHub to run for some robotics projects. In doing so, I've had to work with getting inference to run on an NVIDIA GPU and in this post, I want to highlight my experiences with working with ML modelling libraries and NVIDIA's own tools.
What Works Well
With the growth in machine learning applications in the last ten years, it looks like there's never been a better time for NVIDIA. The NVIDIA CUDA libraries have turned their gaming GPUs into general purpose computing monsters overnight. Vector and matrix operations are crucial to several ML algorithms, and these run several times faster on the GPU than they do on the fastest CPUs. To solidify their niche in this market, the 10- series and newer of NVIDIA GPUs come with dedicated hardware, such as the Deep Learning Accelerator (DLA) and 16-bit floating point (FP16) optimizations, for faster inference performance.
NVIDIA has developed several software libraries to run optimized ML models on their GPUs. The alphabet soup of ML frameworks and their file extensions took me some time to decipher, but the actual workflow of model optimization is surprisingly simple. Once you have a trained your PyTorch or Tensorflow model, you can convert the saved model or frozen checkpoint to the ONNX model exchange format. ONNX lets you convert your ML model from one format to another, and in the case of NVIDIA GPUs, the format of choice is a TensorRT engine plan file. TensorRT engine plan files are bespoke, optimized inference models for a given configuration of CUDA, NVIDIA ML libraries, GPU, maximum batch size, and decimal precision. As such, they are not portable among different hardware/software configurations and they can only be generated on the GPU you want to use for deployment.
When the TensorRT conversion works as it's supposed to, you can expect to see a significant 1.5-3x performance boost over running the Tensorflow/PyTorch model. INT8 models might have even better performance, but this requires a calibration dataset for constructing the TensorRT engine. TensorRT is great for deploying models on the NVIDIA Xavier NX board, since you can also build TensorRT engines which run on the power efficient DLAs as opposed to the GPU.
What Needs Improvement
For all of NVIDIA's good work in developing high performance ML libraries for their GPUs, the user experience of getting started with their ML development software leaves some to be desired. It's imperative to double check all the version numbers of the libraries you need when doing a fresh installation. For instance, a particular version of tensorflow-gpu
only works with a specific point release of CUDA which in turn depends on using the right GPU driver version. All of these need to be installed independently, so there are many sources of error which can result in a broken installation. Installing the wrong version of TensorRT and cuDNN can also result in dependency hell. I am curious to know why all these libraries are so interdependent on specific point releases and why the support for backward compatibility of these libraries is so minimal. One solution around this issue is using one of NVIDIA's several Docker images with all the libraries pre-installed. Occasionally, I also ran into issues with CUDA not initializing properly, but this was fixed by probing for the NVIDIA driver using sudo nvidia-modprobe
.
As an aside, I also found that the accuracy of the TensorRT engine models are subjectively good, but there is noticeable loss in accuracy when compared to running the pretrained Tensorflow model directly. This can be seen here when evaluating the LaneNet road lane detection model:
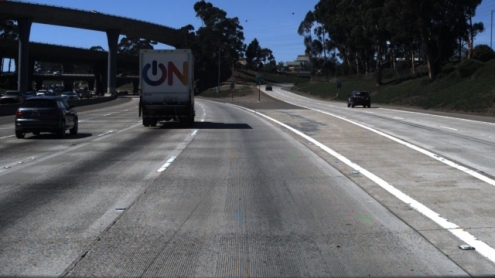
Source Image
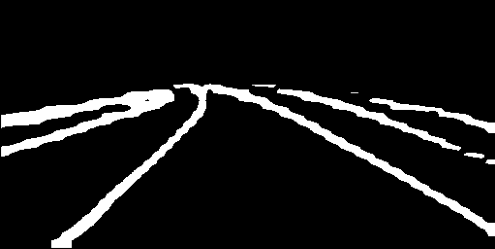
Tensorflow Frozen Checkpoint Inference (80 fps)
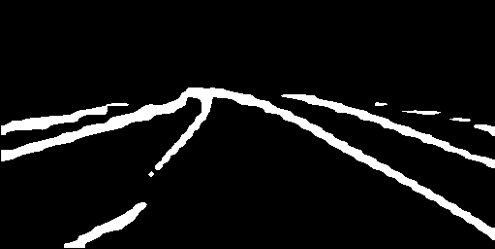
TensorRT engine inference in FP32 mode (144 fps) and FP16 mode (228 fps)
The results are subjectively similar, but there is a clear tradeoff in the accuracy of lane detection and inference time when running these models on the laptop flavour of the NVIDIA RTX 2060.
On the Tensorflow and ONNX side of things, the conversion from a frozen Tensorflow checkpoint file to an ONNX model was more difficult than I anticipated. The tf2onnx
tool requires the names of the input and output nodes for the conversion, and the best way to do this according to the project's GitHub README is to use the summarize_graph
tool which isn't included by default in a Tensorflow distribution. This means downloading and building parts of Tensorflow from source just to check the names of the input and output nodes of the model. Curiously enough, the input and output node names need to be in the format of node:0
, though appending the :0
is not explicitly mentioned in the documentation. I also learned that the .pb
file extension can refer to either a Tensorflow Saved Model or Frozen Model, and both of these cannot be used interchangeably. Luckily, the experience becomes considerably less stressful once the ONNX file is successfully generated and Tensorflow can be removed from the picture. I also tried using the ONNX file with OpenVINO using Intel's DL Workbench Docker image and the conversion to an Intel optimized model was a cinch. That said, the inference performance on an Intel CPU with the OpenVINO model wasn't anywhere close to that on an NVIDIA GPU using a TensorRT model when using the same ONNX model.
Conclusion
I feel it's important to make the setup procedure of DL libraries easier for beginners. The ONNX file format is a good step in the right direction to making things standardized and the optimized model generation software from a variety of hardware manufacturers has been crucial in improving inference performance. The DLAs found on the Jetson boards are quite capable in their own right, while using a fraction of the power a GPU would use. Other vendors have taken note with the Neural Engine debuting with the Apple A11 SoC. Intel has approached the problem at a different angle by adding AVX512 for faster vector operations using the CPU and a Gaussian Neural Accelerator (presumably comparable to a DLA?) with the 10th Gen mobile SoCs. If the last few years is anything to go by, advancements in DL inference performance per watt show no sign of slowing down and it's not hard to foresee a future where a DLA in some shape and form becomes as standardized as the CPU and GPU on most computing platforms.
19 Dec 2020
The visions I had for grad school have not quite been realized during my time at UPenn.
The Statement of Purpose I used for my Masters' admissions was filled with the experiences I had when working on projects on during undergrad. I emphasized that if given the chance, my goal was to advance the state of art in robotics through research. The sentiment was genuine and based on whatever experiences I had in undergrad, I was ready to take a lighter course during my masters to make more time for research. I had pretty much made up my mind to take the thesis option at Penn - granting one with the chance to do original research for two full semesters with a 20% reduction in course load to boot!
However, as I mentioned in my previous posts, my plans changed during my first year. I ended up taking time-consuming courses in my first and second semester which left little time to do research. That's not to say it was for a lack of trying - for I did reach out to a couple of labs located at Pennovation Works, a business incubator and massive robotics research facility under one roof.
I had some good introductory conversations with the passionate PhDs and postdocs at these labs. One of the more notable discussions I had was when a postdoc at one of these labs asked me how I would solve one of the open research problems she was working on. Perplexed by the question, I said that I didn't know enough to answer without reading some papers. After all, I had only just started approaching labs to pursue research and I wasn't even sure where to begin till I got warmed up with the problem.
Ignoring my response, she repeated the question and asked me to try harder. When I was stumped for the second time, she said that I should try to pursue solving the problem with whatever experiences I had in my previous projects. It was a epiphanic moment for me, because till then I had always thought research would require substantial background and theory before building off existing work. It was eye-opening to imagine that solving a difficult problem with whatever I knew was a valid research direction in itself. I left that meeting feeling a lot more certain that I had come to the right place. Unfortunately, a couple of weeks after that meeting, COVID-19 caused the college and all research facilities to close for good. With the burden of courses and new lifestyle changes due to COVID, research was no longer at the top of my priority list and it looked like it would have to wait indefinitely.
In a strange twist of fate, it wasn't for long. My summer internship ended up getting cancelled a few weeks before it was going to start, so to stay busy during the summer, I ended up taking a remote research project at a different lab from the ones I had approached earlier in the year. At first glance, the project I was handed looked like a good fit. It was about creating a autonomous system for quadcopters to avoid midair collisions. I felt like I could leverage some of my previous work to get new ideas. However, soon after I started, the cracks in what I knew started to become apparent. The existing work in the project involved using Model Predictive Control (MPC) and convex optimization for planning collision free trajectories. I only knew about MPC in passing and convex optimization was not a topic I could hope to cover in time for doing anything new in a twelve week project. I would end up spending weeks on what I felt was a novel approach, only to find that the team of PhDs had tried it out without much luck long before. My drawers filled with reams of printed research papers from other university labs working on the same problem. I began rethinking the lab's existing work and it troubled me that the current research direction would only work for a very specific configuration of robots, which as anyone working in robotics knows, is rarely something useful for the real world. At some point, it was clear that the project wasn't a good fit for me, so I dropped out of the lab soon after.
When it comes to original research, I feel master's students are in a middling position as the research work they aspire to do often requires coursework which one only completes near the end of the degree. To that end, I suggest taking advanced versions of courses (such as Model Predictive Control or Adavance Computer Perception) early on. It is important to know the background which a particular research project requires, unless your idea is to solve the problem with what you already know. I also suggest prodding an existing research approach for weaknesses, as new insight can patch the holes in a current direction of the research project. It's also best not to commit early to working on a project, at least not until you have a realistic plan of work for the duration you want to work.
My experience with research was less than ideal, but I would be curious to find out if others have been able to overcome the hurdles which come with trying out a project out of one's depth. Do let me know about your experiences in the comments!
29 Oct 2020
The APStreamline project aims to make streaming live video from companion computers as painless as possible. It was first released as the product of my ArduPilot GSoC project back in 2018. Community feedback for APStreamline was very encouraging and that led me to continue development in 2019. However, there was an issue which had been nagging at me from the outset of its v1 release - adding support for new cameras was tricky due to the confusing coupling of some classes in the codebase. In fact, the most requested feature I've received for APStreamline has been to make it easier to add support for different types of cameras.
APStreamline has grown to support a wide variety of use cases for network adaptive video streaming
The Linux V4L2 driver helps in making it easier to add support for different cameras. Several cameras have a standard interface for capturing video using GStreamer elements. So why does support for each camera need to be baked into the code? The reason is that some cameras use different GStreamer elements, some only support non-standard resolutions, and some even have onboard ASICs for H.264 compression. For example, in the NVIDIA Jetson line of single-board computers have GStreamer pipelines built specifically for accessing the Jetson's ISP and hardware encoder for high quality video encoding. To make the most of each camera and companion computer, it is well worth the effort to add specific support for popular camera models.
All this inspired a rewrite of the code, and I am proud to announce that with the release of APStreamline v2, it is now much simpler to add support for your own camera! Let's first take a look at what devices are already supported:
Cameras Supported
- Logitech C920 webcam
- Raspberry Pi Camera (Raspberry Pi only)
- e-Con AR0521 (requires the Developer Preview version of NVIDIA Jetpack 4.4)
- ZED2 Depth camera (V4L2 mode)
- Any camera which support MJPG encoding (fallback when specific support is not detected. The MJPG stream from the camera is then encoded to H264 using a software encoder.)
Devices Tested
- Raspberry Pi Zero W/2/3B
- NVIDIA Jetson Xavier NX
- x86 computer
Adding Your Own Camera
To add specific your own camera, follow these steps!
1) Figure out the optimized GStreamer pipeline used for your camera. You can usually find this in the Linux documentation for your camera or online developer forums. There are differences for each camera but there is a generic template for most GStreamer pipelines: SRC ! CAPSFILTER ! ENCODER ! SINK
.
There might be more elements or additional capsfilters depending on each camera. In case you aren't sure what to do, feel free to drop a GitHub issue to ask!
2) Create a configuration file for your camera. There are examples in the config/
folder. The element names must match those in the examples for APStreamline to set references to the GStreamer elements correctly.
3) Subclass the Camera
class and override the functions for which you want to add specific support for your camera. To give an example, the ways in which the H264 encoding bitrate is set are different for various cameras and encoder configurations. For instance, pipelines which use the x264enc
encoder can change the bitrate by using g_object_set
, whereas the Raspberry Pi camera GStreamer pipeline needs an IOCTL to change the bitrate.
There is a fair bit of trial and error to discover what the capabilities of a GStreamer pipeline are. Not all pipelines support the dynamic resolution adaptation of APStreamline, so this feature must be disabled if it causes the pipeline to crash in testing.
4) Create a new type for your camera and add it to the CameraType
enum class.
5) Add the enum created in the previous step to the CameraFactory
class.
6) Add a way of identifying your camera to RTSPStreamServer
. A good way of adding a way to identify your camera is by using the Card type property from v4l2-ctl --list-formats-ext --all --device=/dev/videoX
. In case your camera is not specifically detected, the fallback is to encode the MJPG stream from your camera using the H264 software encoder, x264enc
.
7) File a Pull Request to get your camera added to the master branch!
To Do
APStreamline could use your help! Some of the tasks which I want to complete are:
- Add support for the
tegra-video
driver back into the project. Currently this is only supported in APStreamline v1.0, available from the Releases section of the repository
- Update documentation and add more detailed steps for adding a new camera
- Update the APWeb interface to list the actual available resolutions of the camera. Currently it just shows 320x240, 640x480, 1280x720 although the actual camera resolutions may be different
- Switch the APWeb and APStreamline IPC mechanism to using ZeroMQ or rpcgen
- Improve the installation flow. Currently the user needs to run APStreamline from the same directory as its config files for them to be loaded properly. Maybe the configuration files should be moved to
~/.config/?
- Improve the JavaScript in the APWeb interface
- Document the code better!
12 Sep 2020
The repetitive months of quarantine have been exhausting in every single way. One of the sources of light in this unfamiliar tunnel of social isolation has been my new bicycle, on which I have already completed over 1200km in the three odd months of owning it. Philadelphia has relatively flat elevation, good bike lane coverage, and many beautiful trails making it a great city for cycling. There are also good resources on cycling in Philadelphia that may be useful to both the beginner and professional cyclist:
These are some of my favorite spots for cycling in and around Philadelphia. All the places on the below list are easily accessible via dedicated bike lanes from University City and have no entry fees whatsoever.
Ben Franklin Bridge / Penn's Landing
Making another appearance on my blog, the Ben Franklin Bridge
A Philadelphia landmark, the Ben Franklin bridge links the city to Camden, New Jersey. The bridge is flanked by a shared walkway/bike path on both sides, though only the right walkway is presently open. Getting to the highest point of the middle of the bridge can be a drag (and nearly impossible to do when riding a fixed-gear cycle), but it's certainly worth it for the sea breeze and views of the Delaware river adorned by Philadelphia's skyline in the background. One can also hear and feel the periodic vibrations of the NJ Transit train right below the walkway.
Battleship museum at Camden
The Camden Waterfront and the Rutgers Law School are the main attractions on the other side of the bridge. I also stumbled across a floating battleship museum moored on my first trip to the Waterfront.
I personally find Race Street the safest way to go to the Ben Franklin Bridge when starting from the Art Museum. The transition in scenery and smells from the Ben Franklin Parkway to Chinatown is very enjoyable. The Spruce Street corridor makes it easy to go return to University City and it has bollards lining the bike path for additional safety.
Manayunk Trail
Dirt section on the Manayunk Trail
The beginning of this trail is a stone's throw away from the Trek Manayunk showroom, which itself is a short ride from the East Falls bridge. The initial section of the trail consists of wooden boardwalks and some light gravel, followed by miles upon miles of paved, treelined roads running parallel to the Manayunk canal. The number of walking/running pedestrians thins as one goes further down the trail. The flat surface makes it easy to cover long stretches of the trail in quick time. There are only a few greater pleasures than being able to squeeze out a consistent 30+km/hr in top gear. Though I've only made it as far as Bridgeport, the trail goes on till the Valley Forge National Park. A 100km and then a 100 nautical mile round-trip on the Manayunk Trail is currently at the top of my Philadelphia bucket list.
Wissahickon Valley Park
The Wissahickon welcomes one with an amazing view of the Wissahickon Creek
The Wissahickon Valley Park is a short ride away from the East Falls bridge. The park is huge and abundantly green. The first few miles of the trail are on paved roads with small hills. The remainder of the trail is covered with gravel to keep things interesting. I've always liked the feeling of riding on gravel since the lack of purchase on the surface forces a more attentive and focussed riding style. Wissahickon also is home to several mountain biking trails of varying levels of difficulty. I tried some of the mountain biking trails on my hybrid bike, but it soon became apparent that the lack of suspension and the (in mountain bike terms) narrow 35mm tyres made the bike ill-equipped to deal with the off-road features that a proper mountain bike would have taken in stride.
Gravel section of the Wissahickon Trail. A mountain bike would be a lot of fun!
UPenn's Morris Arboretum is only a short ride away from the end of the Wissahickon Park. Entry is free for PennCard holders and the garden has some beautiful sights when the flowers are in season. The entire length of the round-trip when starting from Penn's campus is about 45km to the Arboretum and back.
Martin Luther King Jr Drive / Kelly Drive
Wide roads make brisk cycling feasible
The MLK drive runs along the Schyukill river and it starts near the Philadelphia Art Museum. Normally opened to regular traffic, MLK drive has been closed to motorized vehicles since the start of the pandemic. While the MLK drive can feel crowded with the sheer number of cyclists and runners in the evening, there's plenty of room to ride as fast or slow as you want to. The tall slope near the end of the drive leads to the Strawberry Mansion bridge, which connects to the East side of Fairmount Park.
One of my favourite places to sit down after returning from the above Trails
Kelly Drive lies on the other side of the Schyukill. The views are more scenic, but the path is also much narrower. Kelly Drive sees a lot more pedestrians, so it isn't nearly as smooth as riding on the MLK drive.
Despite the MLK and Kelly Drive often being my first recommendation for new cyclists, I have become disenchanted with going up and down these trails after making several dozen trips to get to Wissahickon and Manayunk.
Cobbs Creek
I have been to Cobbs Creek only once but it's interesting enough to merit a position on this list. The Creek is located in the West part of Philadelphia and extends near the south-west corner of the city. The trail goes in a loop and I liked the gentle slopes which make fast cycling a breeze. However, as the Creek is in a gritty neighborhood, I would suggest making a trip before it gets dark.
11 Jul 2020
Since my last post on COVID19, much has happened. Vaccine development is a topic which I have been following with great interest and it looks like the frontrunner vaccine candidates mentioned in my previous post are still on track for a rollout later this year. I expect we will have a better idea of how things stand after the official release of the Phase I/II data, promised by some of the vaccine biotech companies to come later this month. I will concede that this is the wrong blog for educated opinions on a vaccine, and that you should really follow this one if you're interested in learning more. This particular post is about a different topic which has been on mind for a month.
I have experienced a curious change in my relation to work. My attention span is far less than it what it used to be and there is a considerable drop in productivity as well. It isn't just my work which is affected, for I wasn't even able to bring myself to type out this blog post for nearly a week after creating an empty file with the title. I have tried making progressive steps, such as cutting off the consumption of mental junk food such as social media, but it seems like idling away time on social media was only a small part of the problem.
Ben Franklin Bridge - a good place to get some thinking done
Of course, the COVID19 pandemic and heightened racial tensions in the background have been detrimental to productivity. With the amount of time devoted to being kept up to date on these pressing issues, it's hard to mentally stay on top of things. However, I feel another possible reason for this is that it is harder to find gratification in work anymore. Although this isn't my first remote project, the lack of human contact has made the work feel impersonal and uninspiring. In normal circumstances, a difficult technical problem is an exciting thing to work on, but now it just feels like a mental burden. I have also realized I'm much more willing to work on short term tasks with immediate goals than something which could take weeks to complete. This has made me think that the key issue could be that doing anything which involves delayed gratification is much harder to achieve in these times.
Wikipedia defines delayed gratification as:
Delayed gratification, or deferred gratification, describes the process that the subject undergoes when the subject resists the temptation of an immediate reward in preference for a later reward.
A famous experiment, usually cited in the same breath as this topic, is the Stanford Marshmallow experiment. In this experiment, young children were given the options of 1) receiving one marshmallow immediately 2) receiving two marshmallows if they waited for 15 minutes. This was a long-term study which went on to show that the children who waited longer for two marshmallows had better test scores, social competence, and self-worth as teenagers. Researchers have also found that delayed gratification is a skill well documented in several animals - from chickens to monkeys.
Delayed gratification is a concept which can be applied to work as well - you need to work hard in the moment to experience the satisfaction of seeing a successful project through. However, delayed gratification requires an assurance of stability in the future to be effective. Suppose the children in the marshmallow experiment were told that there was an equal chance of either getting two marshmallows or none at all after waiting for 15 minutes. Would any of them have chosen to wait then?
The main issue which many of us are doubtless experiencing is uncertainty, and with that, any decision making feels uneducated. It's hard to mentally justify working on long-term problems when the near future itself looks unclear. Self-motivation can keep you going for a while, but it is a resource which needs to be topped up regularly. I can definitely see myself being more willing to take risks and go for things which are gratifying in the short-term after the end of this pandemic.
Fortunately, I'm in the position where I can choose my own hours and how much I want to work for week, so I can scale back if I don't enjoy what I'm doing. Some other things which I have been trying out is to keep in touch with my groups of friends and cycling every day to bring some change in environment and structure in my day. Still, I am yearning for one of these days to bring some much needed gratisfaction.