19 Sep 2018
I've read a lot of books in the last two months.
Page for page, I've already far surpassed my 2017 reading throughput this year. Along with my usual cache of fiction and space-flight books, I have read some beautifully written books about cancer and the process of dying such as "When Breath Becomes Air" and "Being Mortal". As much as I hope that advances in medical science will outpace mortality in the next fifty years, death is the only certainty we can contend with in life. I found that reading these books helps me understand what happens at the End Of Life. It's strangely comforting to have a deeper insight into death.
This is what brings me to The Last Lecture, a talk I stumbled upon when binging on Coding Horror.
Dr. Randy Pausch was a professor at CMU and was in the vanguard of the development of virtual reality in the 1990's. Unfortunately, he was diagnosed with pancreatic cancer in 2006. After being told that his cancer had turned terminal, he delivered his final lecture at CMU about "Achieving Your Childhood Dreams" (linked above). It's an incredibly inspirational talk and Randy Pausch is so charismatic that you can't help but to watch the entire video in a single sitting.
Despite the premise, the talk is not about cancer or dying at all. Instead, Dr. Pausch shares anecdotes from his life about he chased his own whimsical childhood dreams.
I loved many parts of this lecture, so I have copied the parts of his excellent book which resonated me the most:
On failure:
Over the years, I also made a point of telling my students that in the entertainment industry, there are countless failed products. It’s not like building houses, where every house built can be lived in by someone. A video game can be created and never make it through research and development. Or else it comes out and no one wants to play it. Yes, video-game creators who’ve had successes are greatly valued. But those who’ve had failures are valued, too—sometimes even more so.
Start-up companies often prefer to hire a chief executive with a failed start-up in his or her background. The person who failed often knows how to avoid future failures. The person who knows only success can be more oblivious to all the pitfalls.
Experience is what you get when you didn’t get what you wanted. And experience is often the most valuable thing you have to offer.
It's a cliché that experience is the best teacher. But, as Randy Pausch would have said, it's a cliché for good reason, because it's so damn true. From one of the most influential talks I've had the pleasure of attending in person, I have to come to realise that failing spectacularly is better than being a mediocre success.
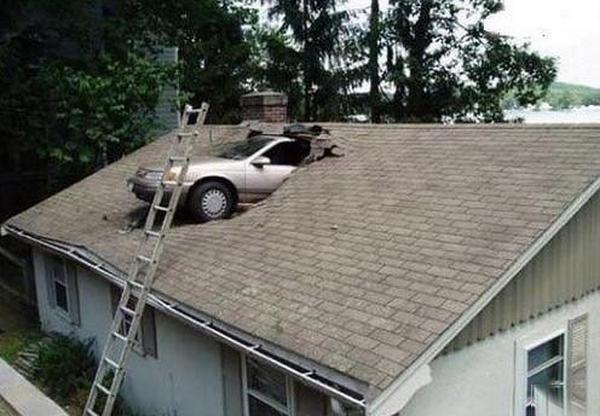
It's a point which I try to shoehorn into every public talk I give to juniors. Sure, you might get laughed at and trampled on for failing terribly, but once you've experienced that, you will realise that failure isn't something to be all that scared about. You'll learn from the mistakes you made when you failed, and eventually, you might even learn to laugh at yourself for failing the way you did.
On brick walls - the insurmountable obstacles that stop you from getting what you want:
The brick walls are there to stop the people who don’t want it badly enough. They’re there to stop the other people.
There's a lot of meaning in those two sentences and it has really changed my perception of "not taking no for an answer". The "other people" refers to those who don't have the tenacity or the zeal to achieve their childhood dreams. The brick walls are a test for seeing if you're really sure that you want something, be it a degree, a job, or maybe a way to finance your latest hobby. I feel like a lot of challenging decisions in life can become a lot clearer after perceiving it this way. If you don't find the motivation to attempt climbing the brick wall between you and your dreams, then maybe those dreams weren't yours in the first place.
On the ultimate purpose of teachers:
In the end, educators best serve students by helping them be more self-reflective. The only way any of us can improve—as Coach Graham taught me—is if we develop a real ability to assess ourselves. If we can’t accurately do that, how can we tell if we’re getting better or worse?
Self-reflection and introspection are very underrated. It's easy to reduce education to a commercial service (only more true these days than ever) and teachers as the providers. But, education is something of a "head fake". As Randy Pausch describes it, head fakes are a type of indirect learning, often more important than the content of what is being taught. He elaborates on this by saying:
When we send our kids to play organized sports—football, soccer, swimming, whatever—for most of us, it’s not because we’re desperate for them to learn the intricacies of the sport.
What we really want them to learn is far more important: teamwork, perseverance, sportsmanship, the value of hard work, an ability to deal with adversity.
I can't remember the conditions needed for each oxidation state of Manganese, but I do remember learning how to learn and how to get through some very sticky situations. Most of the benefits I've gleaned from studying from school are less quantifiable than a number of exam results, but I wouldn't trade those life lessons for anything. It's this kind of meta education which I find sorely lacking at BITS. I miss the deeper perspectives on my subjects which made me question the way things are. Fiercely independent college students may not need the same hand-holding as naive school students, but the need for some kind of guidance is just as necessary.
On hard work:
A lot of people want a shortcut. I find the best shortcut is the long way, which is basically two words: work hard.
As I see it, if you work more hours than somebody else, during those hours you learn more about your craft. That can make you more efficient, more able, even happier. Hard work is like compounded interest in the bank. The rewards build faster.
On apologising to people:
Apologies are not pass/fail. I always told my students: When giving an apology, any performance lower than an A really doesn’t cut it.
Halfhearted or insincere apologies are often worse than not apologizing at all because recipients find them insulting. If you’ve done something wrong in your dealings with another person, it’s as if there’s an infection in your relationship. A good apology is like an antibiotic; a bad apology is like rubbing salt in the wound.
07 Sep 2018
I love videogames. I can't call myself a serious gamer, but I've played my share of good and bad games ever since I started playing Super Mario Advance 4 on a GBA SP fourteen years ago. Some games have left a deeper impression on me than others. Objectively, the games in this list aren't necessarily the best I have ever played, but they are the ones with which I've made the most memories.
Microsoft Flight Simulator 2004 (PC)
Released in 2003. Played from 2005 - 2013.
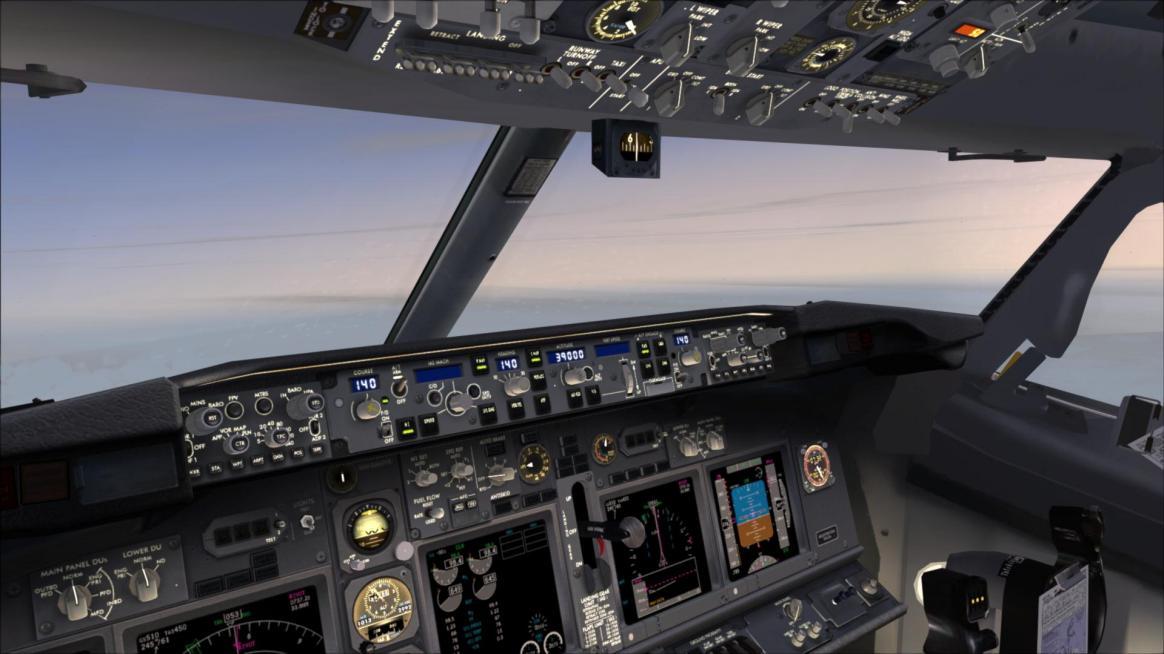
This is the first major PC game I've played. My dad got this for me with a Logitech Extreme 3D Pro joystick on one of his business trips. Eight year old me was blown away by the packaging of the game - it came with several booklets in a box, and the game itself was split in as many as four disks! I fondly remember being awed at the number of pre-loaded airplanes, the 3D graphics (and gorgeous virtual cockpits!), and how hellishly difficult it was to play on our vintage 1998 desktop. The poor desktop's Pentium 3 CPU could only just sputter out 5-6 fps at the lowest settings on a good day. Not to mention that the game's relatively enormous 2.5 GB installation size took up more than half of the computer's 5GB HDD. Many years later, I realised that dealing with these absurd hardware limitations is what got me into computers in the first place.
Nevertheless, my desktop's measly hardware hardly deterred the tenacious pilot in me. I enjoyed piloting small aircraft like the Piper Cub just as much as I enjoyed flying the 747-400. There was something so very satisfying in landing a plane safely with the sun creeping over the horizon at dawn. It didn't take me long to find the very active modding scene for FS2004 on websites like Simviation.com. Several add-ons are still actively developed to this day.
However, it wasn't until we got a new computer in 2008 that I really got to see the game for what it was. The new computer was more than capable of pushing out 60 fps in Ultra High quality. Scenery details, water shaders, and even the runway textures had a photo-realistic effect to them. I scoured every glitched nook and cranny of the Flight Simulator world. I mastered the art of flaring commercial aircraft before landing and taxiing it back to the terminal gate.
At this point, my add-on collection had swollen to over 10GB, nearly four times as large as the original game. There were several oddballs in my collection - including a Mitsubishi Pajero, an Asuka Cruise Ship, and a Bald Eagle which flew like a glider for all intents and purposes. My favourites among my add-on collection were the MD-9, DC-10, OpenSky 737-800, XB-70 Valkyrie, and a an amazing Concorde pack complete with dozens of different liveries. I also liked flying the Tupelov Tu-95 and the Lockheed U-2 "Dragon Lady". Alas, I couldn't shift my joystick setup after moving to college. Had I been able to, there is a good chance I would still be playing it - fifteen years after the game's initial release.
Need For Speed: Most Wanted (PC)
Released in 2005. Played from 2008 - 2011.
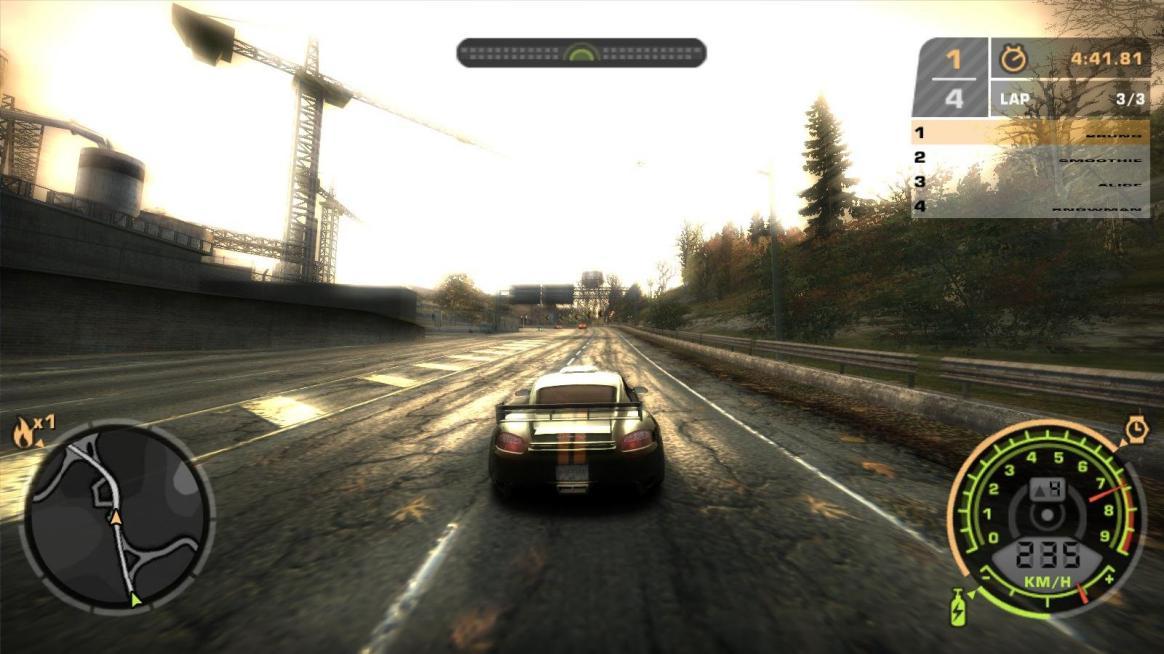
The only racing game in this list and my favourite overall by far. NFSMW won't win any awards for realism in its driving physics (go play DiRT if you're a stickler for realism), but it certainly will for mindless fun. There is so much that EA got right with this game - the fresh, overbright visuals, excellent world design with plenty of shortcuts and obstacles to crush unsuspecting cop cars, a good progression system, and a wicked smart cop AI to make cop chases true white-knuckle affairs.
The storyline is simple and the game has plenty of expository cutscenes to make you hate your main rival, Clarence "Razor" Callahan for stealing your brand-new BMW M3 GTR. There are a total of fifteen bosses. On beating each boss, you get a chance to "steal" their uniquely styled car through a lottery system. Winning a boss's car made it much easier to progress through the game. Each car in the game's roster can be customised in every aspect and I spent several hours tuning and buying parts for all the cars in my garage to suit my playing style.
The sheer excitement in cop chases is something I haven't found in any NFS game I've played since MW. On maxing out a car's "Heat", the cops' cars would be upgraded from the bovine Ford Crown Victoria to the absurdly fast Chevy Corvette. Being able to tap on to the police chatter was a superb game mechanic, letting one plan their way around road-blocks and spike-traps. The game also offered plenty of room for creativity to get out of tight situations in cop chases. Lastly, beating Razor at the end is right up there with the most badass moments I've ever had in a videogame.
I did play a handful of NFS games after MW, but they just fell flat on the fun aspect which MW had in droves. NFS ProStreet brought car damage and photo-realistic graphics, but the serious racing style was a huge tonal shift from the absurd shenanigans of MW. NFS Shift was cut from the same cloth as ProStreet. Hot Pursuit looked like a return to form for EA, but sadly it couldn't live up to my expectations in gameplay, which just felt clunky compared to MW. I do look forward to playing the 2012 remake of MW to see if it can replicate the charm of the first one.
Pokemon Black (DS)
Released in 2011. Played from 2011 - 2014.
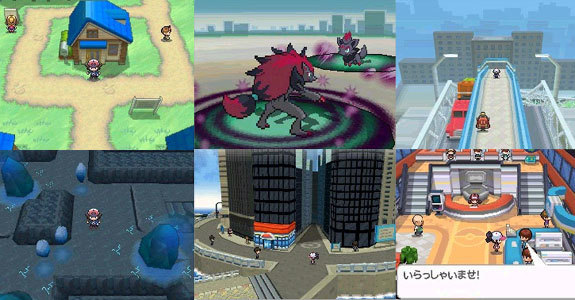
Pokemon Black is not the first Pokemon game I've played, but it is the first I have played on a Nintendo console after rekindling my love for Pokemon. Coming off playing Platinum (which I had also enjoyed), Pokemon Black was a breath of fresh air. For perhaps the only time in the series' history, GameFreak took a number of risks, most of which paid off. In Black & White only brand-new Unova Pokemon are available in the wild before beating the Elite Four. This forced players to learn the game's new mechanics and adopt new battling strategies. The visuals were the best I had ever seen from a DS game with lovingly animated Pokemon sprite-work in the battles. Even the storyline was actually thought provoking and it was far better than the "Ten Year Old Beats Team After World Domination\" narrative that had been done to death in the earlier games.
The overworld was rich and varied and the game's season mechanic changed the appearance of several areas over the course of a year. The music was fantastic in several places and a major step up from the Gen IV themes.
Being a Pokemon game, it wasn't completely free of hand-holding, but at least it was far better than Sun & Moon which came half a decade after the release of Black & White. My major gripe with BW is that Unova is much too linear and literally forces you halfway around the map to reach the Elite Four.
However, the regular gameplay was just a distraction for the wonderful Competitive Battling scene. This was the first Pokemon game in which I put my new-found knowledge of EVs and IVs to fruition. The Gen V Wi-Fi Competitive Battling scene was amazing thanks to Team Preview, BattleSpot, and International Tourneys held online every few months. Thanks to RNG manipulation and the help of an equally geeky friend, I managed to breed a full team of 6x31 IV Pokemon with ideal Natures, all obtained through completely legal means. I discovered my love of Double Battles through the International Tourneys, which I still maintain is the best way to play Pokemon. My go to team in the Tourneys was an immensely bulky Tailwind Suicune with a wall-breaking Life Orb Gengar, backed up by a Physical Fighting Gem Virizion, and a Toxic Orb Gliscor or a Sitrus Berry Gyarados for shutting down any physical attackers. This unconventional team checked a wide variety of the common suspects in Gen V Doubles, most importantly the nearly omnipresent Rotom-W.
Pokemon also gave me my first taste of online community. I joined Smogon in early 2012. My Smogon username still perpetuates in every onlineaccount I've made since then. I watched the birth of the Gen V metagame with my own eyes. Movesets, suspect tests, and analyses were uploaded and discussed at a furious pace on the Dragonspiral Tower sub-forum. Through briefly working for Smogon's C&C team and #grammar
on Smogon IRC, I made some great online friends, some of whom I still speak with to this day. The community was warm and supportive. It's one of the many things I can fondly remember being a part of back in 2012.
After Pokemon Black, I played Pokemon Alpha Sapphire in late 2015. AS was a delightful blast from the past and it fully satiated my decade-long nostalgia for Hoenn. This was in no small part thanks to the post-game Delta Episode which is one of my favourite sequences of any Pokemon game.
About a year later, I picked up Pokemon Moon but I could not get into it at all. I was thoroughly underwhelmed by the constant hand-holding which severely detracted from the core experience of the game. My aging 3DS died at about the same time I finished it, marking a fitting end to my relationship with the series for the time being. Here's hoping Gen VIII on the Switch is truly a special experience which Pokemon players have been missing since Black came out.
17 Aug 2018
Download APSync with APStreamline built in from here (BETA)!
I'm very excited to announce the release of a network adaptive video streaming solution for ArduPilot!
Links to the code
About
The APSync project currently offers a basic video streaming solution for the Raspberry Pi camera. APStreamline aims to complement this project by adding several useful features:
-
Automatic quality selection based on bandwidth and packet loss estimates
-
Selection of network interfaces to stream the video
-
Options to record the live-streamed video feed to the companion computer
-
Manual control over resolution and framerates
-
Multiple camera support using RTSP
-
Hardware-accelerated H.264 encoding for the Raspberry Pi
-
Camera settings configurable through the APWeb GUI
I'm chuffed to say that this has not only met the requirements for the GSoC project but has also covered the stretch goals I had outlined in my proposal!
Streaming video from robots is an interesting problem. There are several different use cases – you might be a marine biologist with a snazzy BlueROV equipped with several cameras or a UAV enthusiast with the itch of flying FPV. APStreamline caters to these and several other use cases.
While APStreamline works on the all network interfaces available on the Companion Computer (CC), its main value lies in the case of streaming on unreliable networks such as Wi-Fi as in most cases, we use the Companion Computer (CC) in Wi-Fi hotspot mode for streaming the video. Due to the limited range of 2.4GHz Wi-Fi, the Quality-of-Service (QoS) progressively gets worse when the robot moves further away from the receiving computer.
This project aims to fixes problem by dynamically adjusting the video quality in realtime. Over UDP we can obtain estimates of QoS using RTCP packets received from the receiver. These RTCP packets provide helpful QoS information (such as RTT and packet loss) which can be used for automatically changing the bitrate and resolution of the video delivered from the sender.
Running the Code
Hardware
All the following instructions are for installing APStreamline and APWeb on the CC. A Raspberry Pi 2/3/3B+ with the latest version of Raspian or APSync is a good choice. Intel NUC's are excellent choices as well.
Do note that the Raspberry Pi 3 and 3B+ have very low power Wi-Fi antennae which aren't great for video streaming. Using a portable Wi-Fi router like the TPLink MR-3020 can dramatically improve range. Wi-Fi USB dongles working in hotspot mode can help as well.
Installing APStreamline
Install the gstreamer
dependencies:
sudo apt install libgstreamer-plugins-base1.0* libgstreamer1.0-dev libgstrtspserver-1.0-dev gstreamer1.0-plugins-bad gstreamer1.0-plugins-ugly python3-pip
Install meson
from pip
and ninja
for building the code:
sudo pip3 install meson
sudo apt install ninja-build
Navigate to your favourite folder folder and run:
git clone -b release https://github.com/shortstheory/adaptive-streaming
meson build
cd build
sudo ninja install # installs to /usr/local/bin for APWeb to spawn
On the Raspberry Pi, use sudo modprobe bcm2835-v4l2
to load the V4L2 driver for the Raspberry Pi camera. Add bcm2835-v4l2
to /etc/modules
for automatically loading this module on boot.
Installing APWeb
The APWeb server project enables setting several flight controller parameters on the fly through the use of a Companion Computer (e.g. the Raspberry Pi). We use this APWeb server for configuring the video streams as well.
Clone the forked branch with APStreamline support here:
git clone -b video_streaming https://github.com/shortstheory/APWeb.git
cd APWeb
Install libtalloc-dev
and get the MAVLink submodule:
sudo apt-get install libtalloc-dev
git submodule init
git submodule update
Build APWeb:
cd APWeb
make
./web_server -p 80
In case it fails to create the TCP socket, try using sudo ./web_server -p 80
. This can clearly cause bad things to happen so be careful!
Usage
Video livestreams can be launched using RTSP. It is recommended to use RTSP for streaming video as it provides the advantages of supporting multiple cameras, configuring the resolution on-the-fly, and recording the livestreamed video to a file.
APWeb
Start the APWeb server. This will serve the configuration page for the RTSP stream server. Connect to the web server in your favourite web browser by going to the IP address of the Companion Computer.
On navigating to the new video/
page, you will be presented with a page to start the RTSP Server:
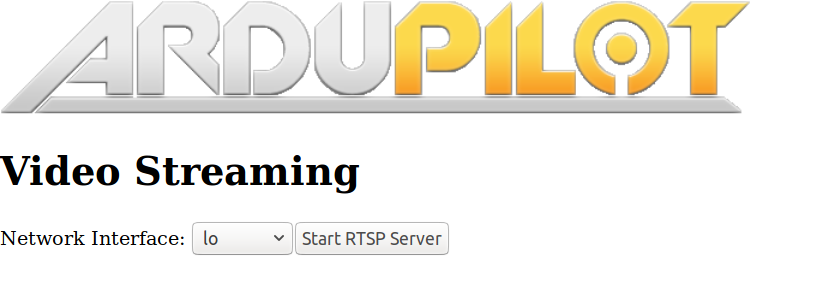
On selecting the desired interface and starting the RTSP Server, the APWeb server will spawn the Stream Server process. The stream server will search for all the V4L2 cameras available in /dev/
. It will query the capabilities of all these cameras and select hardware encoding or software encoding accordingly. The list of available cameras can be refreshed by simply stopping and starting the server.
From here, the APWeb page will display the list of available RTSP streams and their mount points:
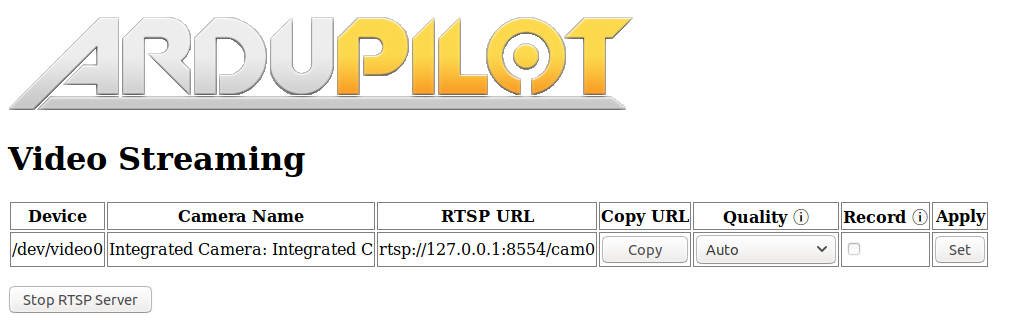
The video quality can either be automatically set based on the available network bandwidth or set manually for more fine-grained control.
The APWeb page also presents an option to record the video stream to a file on the CC. For this the video stream must be opened on the client. This works with any of the manually set resolutions but does not work with Auto quality. This is because the dynamically changing resolution causes problems with the file recording pipeline. An exception to this is the UVC cameras which can record to a file in Auto mode as well.
The RTSP streams can be viewed using any RTSP player. VLC is a good choice. Some GCS such as QGroundControl and Mission Planner can also stream video over RTSP.
For example, this can be done by going to "Media > Open Network Stream" and pasting in the RTSP Mount Point for the camera displayed in the APWeb configuration page. However, VLC introduces two seconds of latency for the jitter reduction, making it unsuitable for critical applications. To circumvent this, RTSP streams can also be viewed at lower latency by using the gst-launch
command:
gst-launch-1.0 playbin uri=<RTSP-MOUNT-POINT> latency=100
As an example RTSP Mount Point looks like: rtsp://192.168.0.17:8554/cam0
. Refer to the APWeb page to see the mount points given for your camera.
Standalone
Launch the RTSP stream server by running:
stream_server <interface>
The list of available network interfaces can be found by running ifconfig
. Streams can be connected to using gst-launch
.
Things to Try Out
-
Use a variety of different cameras and stream several at the same time
-
Try recording the live-streamed video to a file on the CC
-
Play with the Auto quality streaming feature on different types of network interfaces
22 Jul 2018
Much time has passed and much code has been written since my last update. Adaptive Streaming (a better name TBD) for Ardupilot is nearly complete and brings a whole slew of features useful for streaming video from cameras on robots to laptops, phones, and tablets:
- Automatic quality selection based on bandwidth and packet loss estimates
- Options to record the live-streamed video feed to the companion computer (experimental!)
- Fine tuned control over resolution and framerates
- Multiple camera support over RTSP
- Hardware-accelerated H.264 encoding for supported cameras and GPUs
- Camera settings configurable through the APWeb GUI
Phew!
The configuration required to get everything working is minimal once the required dependencies have been installed. This is in no small part possible thanks to the GStreamer API which took care of several low level complexities of live streaming video over the air.
Streaming video from aerial robots is probably the most difficult use case of Adaptive Streaming as the WiFi link is very flaky at these high speeds and distances. I've optimised the project around my testing with video streaming from quadcopters so the benefits are passed on to streaming from other robots as well.
Algorithm
I've used a simplification of TCP's congestion control algorithm for Adaptive Streaming. I had looked at other interesting approaches including estimating receiver buffer occupancy, but using this approach didn't yield significantly better results. TCP's congestion control algorithm avoids packet loss by mandating ACKs for each successfully delivered packet and steadily increasing sender bandwidth till it reaches a dynamically set threshold.
A crucial difference for Adaptive Streaming is that 1) we stream over UDP for lower overhead (so no automatic TCP ACKs here!) 2) H.264 live video streaming is fairly loss tolerant so it's okay to lose some packets instead of re-transmitting them.
Video packets are streamed over dedicated RTP packets and Quality of Service (QoS) reports are sent over RTCP packets. These QoS reports give us all sorts of useful information, but we're mostly interested in seeing the number of packets loss between RTCP transmissions.
On receiving a RTCP packet indicating any packet loss, we immediately shift to a Congested State (better name pending) which significantly reduces the rate at which the video streaming bandwidth is increased on receiving a lossless RTCP packet. The encoding H.264 encoding bitrate is limited to no higher than 1000kbps in this state.
Once we've received five lossless RTCP packets, we shift to a Steady State which can encode upto 6000kbps. In this state we also increase the encoding bitrate at a faster rate than we do in the Congested State. A nifty part of dynamically changing H.264 bitrates is that we can also seamlessly switch the streamed resolution according to the available bandwidth, just like YouTube does with DASH!
This algorithm is fairly simple and wasn't too difficult to implement once I had figured out all the GStreamer plumbing for extracting packets from buffers. With more testing, I would like to add long-term bitrate adaptations for the bitrate selection algorithm.
H.264 Encoding
This is where we get into the complicated and wonderful world of video compression algorithms.
Compression algorithms are used in all kinds of media, such as JPEG for still images and MP3 for audio. H.264 is one of several compression algorithms available for video. H.264 takes advantage of the fact that a lot of the information in video between frames is redundant, so instead of saving 30 frames for 1 second of 30fps video, it saves one entire frame (known as the Key Frame or I-Frame) of video and computes and stores only the differences in frames with respect to the keyframe for the subsequent 29 frames. H.264 also applies some logic to predict future frames to further reduce the file size.
This is by no means close to a complete explanation of how H.264 works, for further reading I suggest checking out Sid Bala's explanation on the topic.
The legendary Tom Scott also has a fun video explaining how H.264 is adversely affected by snow and confetti!
The frequency of capturing keyframes can be set by changing the encoder parameters. In the context of live video streaming over unstable links such as WiFi, this is very important as packet loss can cause keyframes to be dropped. Dropped keyframes severely impact the quality of the video until a new keyframe is received. This is because all the frames transmitted after the keyframe only store the differences with respect to the keyframe and do not actually store a full picture on their own.
Increasing the keyframe interval means we send a large, full frame less frequently, but also means we would suffer from terrible video quality for an extended period of time on losing a keyframe. On the other hand, shorter keyframe intervals can lead to a wastage of bandwidth.
I found that a keyframe interval of every 10 frames worked much better than the default interval of 60 frames without impacting bandwidth usage too significantly.
Lastly, H.264 video encoding is a very computationally expensive algorithm. Software-based implementations of H.264 such as x264enc
are well supported with several configurable parameters but have prohibitively high CPU requirements, making it all but impossible to livestream H.264 encoded video from low power embedded systems. Fortunately, the Raspberry Pi's Broadcom BCM2837 SoC has a dedicated H.264 hardware encoder pipeline for the Raspberry Pi camera which drastically reduces the CPU load in high definition H.264 encoding. Some webcams such as the Logitech C920 and higher have onboard H.264 hardware encoding thanks to special ASIC's dedicated for this purpose.
Adaptive Streaming probes for the type of encoding supported by the webcam and whether it has the IOCTL's required for changing the encoding parameters on-the-fly.
H.264 has been superseded by the more efficient H.265 encoding algorithm, but the CPU requirements for H.265 are even higher and it doesn't enjoy the same hardware support as H.264 does for the time being.
GUI
The project is soon-to-be integrated with the APWeb project for configuring companion computers. Adaptive Streaming works by creating an RTSP Streaming server running as a daemon process. The APWeb process connects to this daemon service over a local socket to populate the list of cameras, RTSP mount points, and available resolutions of each camera.
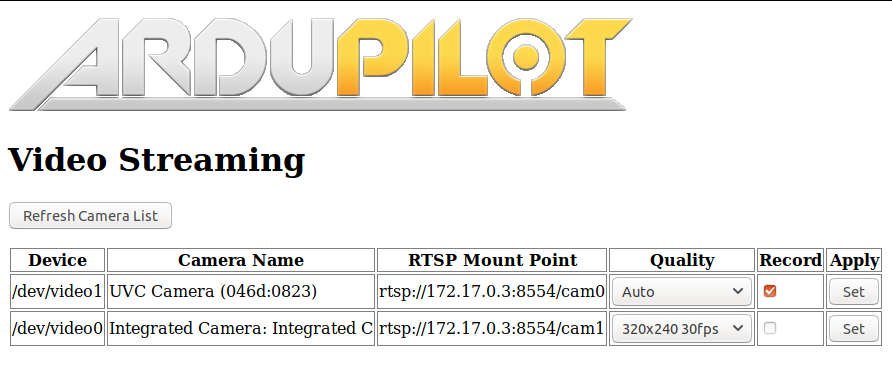
The GUI is open for improvement and I would love feedback on how to make it easier to use!
Once the RTSP mount points are generated, one can livestream the video feed by entering in the RTSP URL of the camera into VLC. This works on all devices supporting VLC. However, VLC does add two seconds of latency to the livestream for reducing the jitter. I wasn't able to find a way to configure this in VLC, so an alternative way to get a lower latency stream is by using the following gst-launch
command in a terminal:
gst-launch-1.0 playbin uri=<RTSP Mount Point> latency=100
In the scope of the GSoC timeline, I'm looking to wind down the project by working on documentation, testing, and reducing the cruft from the codebase. I'm looking forward to integrating this with companion repository soon!
Links to the code
https://github.com/shortstheory/adaptive-streaming
https://github.com/shortstheory/APWeb
Title image from OscarLiang
05 Jun 2018
I'm really excited to say that I'll be working with Ardupilot for the better part of the next two months! Although this is the second time I'm making a foray into Open Source Development, the project at hand this time is quite different from what I had worked on in my first GSoC project.
Ardupilot is an open-source autopilot software for several types of semi-autonomous robotic vehicles including multicopters, fixed-wing aircraft, and even marine vehicles such as boats and submarines. As the name suggests, Ardupilot was formerly based on the Arduino platform with the APM2.x flight controllers which boasted an ATMega2560 processor. Since then, Ardupilot has moved on to officially supporting much more advanced boards with significantly better processors and more robust hardware stacks. That said, these flight controllers contain application specific embedded hardware which is unsuitable for performing intensive tasks such as real-time object detection or video processing.
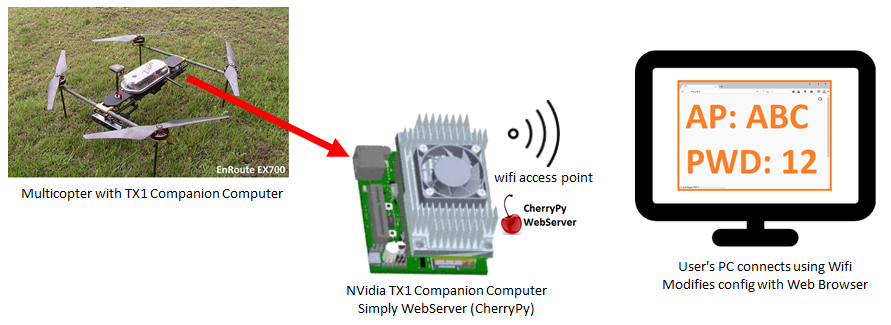
CC Setup with a Flight Computer
APSync is a recent Ardupilot project which aims to ameliorate the limited processing capability of the flight controllers by augmenting them with so-called companion computers (CCs). As of writing, APSync officially supports the Raspberry Pi 3B(+) and the NVidia Jetson line of embedded systems. One of the more popular use cases for APSync is to enable out-of-the-box live video streaming from a vehicle to a laptop. This works by using the CC's onboard WiFi chip as a WiFi hotspot to stream the video using GStreamer. However, the current implementation has some shortcomings which are:
- Only one video output can be unicasted from the vehicle
- The livestreamed video progressively deteriorates as the WiFi link between the laptop and the CC becomes weaker
This is where my GSoC project comes in. My project is to tackle the above issues to provide a good streaming experience from an Ardupilot vehicle. The former problem entails rewriting the video streaming code to allow for sending multiple video streams at the same time. The latter is quite a bit more interesting and it deals with several computer networks and hardware related engineering issues to solve. "Solve" is a subjective term here as there isn't any way to significantly boost the WiFi range from the CC's WiFi hotspot without some messy hardware modifications.
What can be done is to degrade the video quality as gracefully as possible. It's much better to have a smooth video stream of low quality than to have a high quality video stream laden with jitter and latency. At the same time, targeting to only stream low quality video when the WiFi link and the processor of the CC allows for better quality is inefficient. To "solve" this, we would need some kind of dynamically adaptive streaming mechanism which can change the quality of the video streamed according to the strength of the WiFi connection.
My first thought was to use something along the lines of Youtube's DASH (Dynamically Adaptive Streaming over HTTP) protocol which automatically scales the video quality according to the available bandwidth. However, DASH works in a fundamentally different way from what is required for adaptive livestreaming. DASH relies on having the same video pre-encoded in several different resolutions and bitrates. The server estimates the bandwidth of its connection to the client. On doing so, the server chooses one of the pre-encoded video chunks to send to the client. Typically, the server tries to guess which video chunk can deliver the best possible quality without buffering.
Youtube's powerful servers have no trouble encoding a video several times, but this approach is far too intensive to be carried out on a rather anemic Raspberry Pi. Furthermore, DASH relies on QoS (short for Quality of Service which includes parameters like bitrate, jitter, packet loss, etc) reports using TCP ACK messages. This causes more issues as we need to stream the video down using RTP over UDP instead of TCP. The main draw of UDP for livestreaming is that performs better than TCP does on low bandwidth connections due to its smaller overhead. Unlike TCP which places guarantees on message delivery through ACKs, UDP is purely best effort and has no concept of ACKs at the transport layer. This means we would need some kind of ACK mechanism at the application layer to measure the QoS.
Enter RTCP. This is the official sibling protocol to RTP which among other things, reports packet loss, cumulative sequence number received, and jitter. In other words - it's everything but the kitchen sink for QoS reports for multimedia over UDP! What's more, GStreamer natively integrates RTCP report handling. This is the approach I'll be using for getting estimated bandwidth reports from each receiver.
I'll be sharing my experiences with the H.264 video encoders and hardware in my next post.